You enter a contest. A million dollars is at stake. Forty-one thousand teams from 186 different countries are clamoring for the prize and the glory. You edge into the top 5 contestants, but there is only one prize, and one winner. Second place is the first loser. What do you do?
Team up with the winners, of course.
The Netflix Prize is a competition that is awarding $1,000,000 to whomever can come up with the best improvement to their movie recommendation engine. Their system looks at the massive amounts of movie rental data to try to predict how well users will like other movies. For example, if you like Coraline, you may also like Sweeney Todd. But Netflix’s recommendation engine isn’t great at making predictions, so they decided to offer a bounty to anyone who could come up with a system that has a verifiable 10% improvement to Netflix’s prediction accuracy.
The contest recently ended with two teams jockeying for the prize. During the two and a half years the contest has been active, several individuals and small groups dominated the contest leaderboard;, with competition among 41,000 teams from 186 different countries. The competition became fierce, resulting in coalitions forming. The team “BellKor’s Pragmatic Chaos” formed from the separate teams “BellKor” (part of the Statistics Research Group in AT&T labs), “BigChaos” (a group of folks who specialize in building recommender systems), and “PragmaticTheory” (two Canadian engineers with no formal machine learning or mathematics training). Another conglomerate team, “The Ensemble“, is made up of “Grand Prize Team” (itself a coalition of members combining strategies to win the prize), “Vandelay Industries (another mish-mash of volunteers)”, and “Opera Solutions“.
;
At first, it looked like BellKor’s Pragmatic Chaos won. But now it looks like The Ensemble won. Netflix says it will verify and announce the winner in a few weeks.
Who the hell cares? Why is this interesting in the slightest? Ten percent seems so insignificant.
Well, predicting human behavior seems impossible. But this contest has clearly shown that some amount of improvement in prediction of complicated human behavior is indeed possible. And what’s really interesting about the winning teams is that no single machine learning or statistical technique dominates by itself. Each of the winning teams “blends” a lot of different approaches into a single prediction engine.
Artificial neural networks. Singular value decomposition. Restricted Boltzmann Machines. K-Nearest Neighbor Algorithms. Nonnegative matrix factorization. These are all important algorithms and techniques, but they aren’t best in isolation. Blending is key. Even the teams in the contest were blended together.
United we stand.
Each technique has its strengths and weaknesses. Where one predictor fails, another can take up the slack with its own unique take on the problem.
BellKor, in their 2008 paper describing their approach, made the following conclusions about what was important in making predictions:
- Movies are selected deliberately by users to be ranked. The movies are not randomly selected.
- Temporal effects:
- Movies go in and out of popularity over time.
- User biases change. For example, a user may rate average movies “4 stars”, but later on decide to rate them “3 stars”.
- User preferences change. For example, a user may like thrillers one year, then a year later become a fan of science fiction.
- Not all data features are useful. For example, details about descriptions of movies were significant, and explained some user behaviors, but did not improve prediction accuracy.
- Matrix factorization models were very popular in the contest. Variations of these models were very accurate compared to other models.
- Neighborhood models and their variants were also popular.
- For this problem, increasing the number of parameters in the models resulted in more accuracy. This is interesting, because usually when you add more parameters, you risk over-fitting the data. For example, a naive algorithm that has “shoe color” as an input parameter might see a bank that was robbed by someone wearing red shoes, and conclude that anyone wearing red shoes was a potential bank robber. For another classic example of over-fitting, see the Hidenburg Omen.
- To make a great predictive system, use a few well-selected models. But to win a contest, small incremental improvements are needed, so you need to blend many models to refine the results.
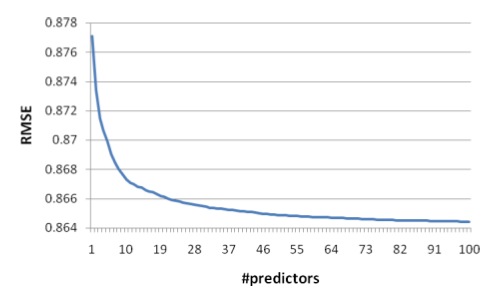
RMSE (error) goes down as the number of blended predictors goes up. But the steepest reduction in error happens with only a handful of predictors — the rest of them only gradually draw down the error rate.
Yehuda Koren, one of the members of BellKor’s Pragmatic Chaos and a researcher for Yahoo! Israel, went on to publish another paper that goes into more juicy details about their team’s techniques.
I hope to see more contests like this. The KDD Cup is the most similar one that comes to mind. But where is the ginormous cash prize???
3 responses to “United we stand”